
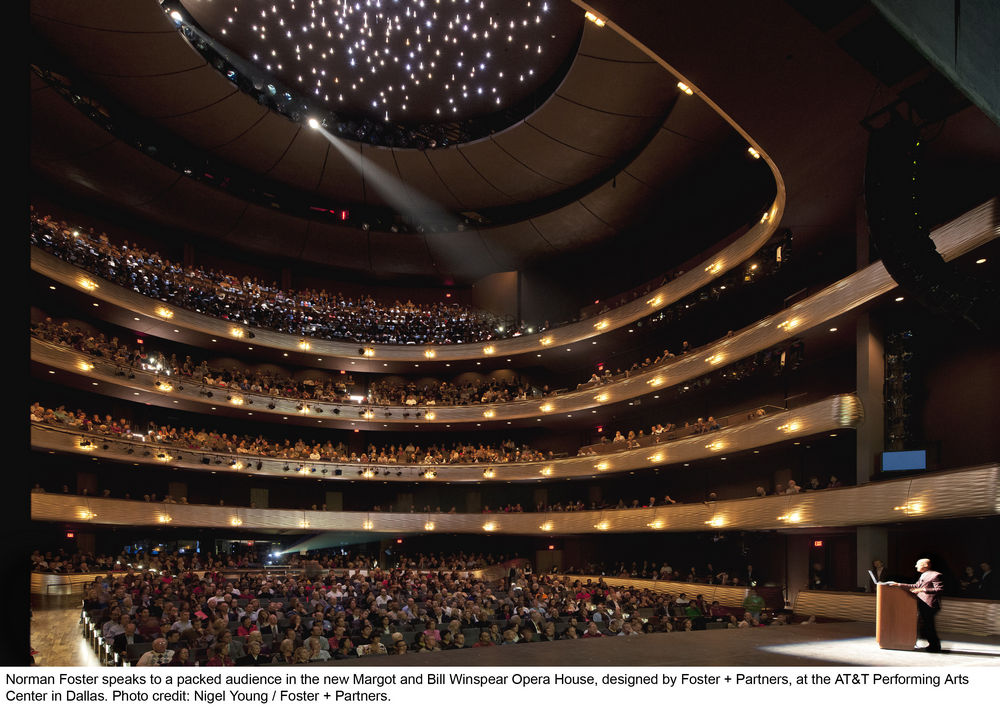
This approach tries to reduce the redundancy in the Walker approach by dividing the regional coverage into several latitude regions that can be addressed by different pairs of satellites’ altitude and inclination, allowing for a better revisit time or a reduced number of satellites.īesides the analytic methods, the genetic algorithm (GA) is known for its robustness in obtaining a global optimum solution for nonlinear multivariable problems through its stochastic and heuristic search algorithms. Another approach to optimize a satellite constellation design by using tiers of satellites with variations in the orbit’s altitude and inclination parameters was proposed Razoumny et al. In the process, a satellite’s life cycle cost and its capacity is traded off to satisfy the minimum per-channel performance requirement. The idea of staged deployments of satellite constellations in LEO was also proposed by including the uncertainty feature of the expected number of users and activity level. Indrikis and Cleave, in their work about SPACE EGGS or the Satellite Coverage Model for LEO (Low Earth Orbit) Constellations, also tried to access the effectiveness of global, regional, and area coverage for proliferated small satellite constellations in low altitude orbits, stressing the capability of conventional analytical techniques. Related to the coverage mission, the idea of satellite constellation designs for complex coverage was presented by Ulybyshev. Besides, a ground track-based approach has been developed, and recently the sliding ground track concept applied to constellations composed of one or more orbital planes has been introduced.
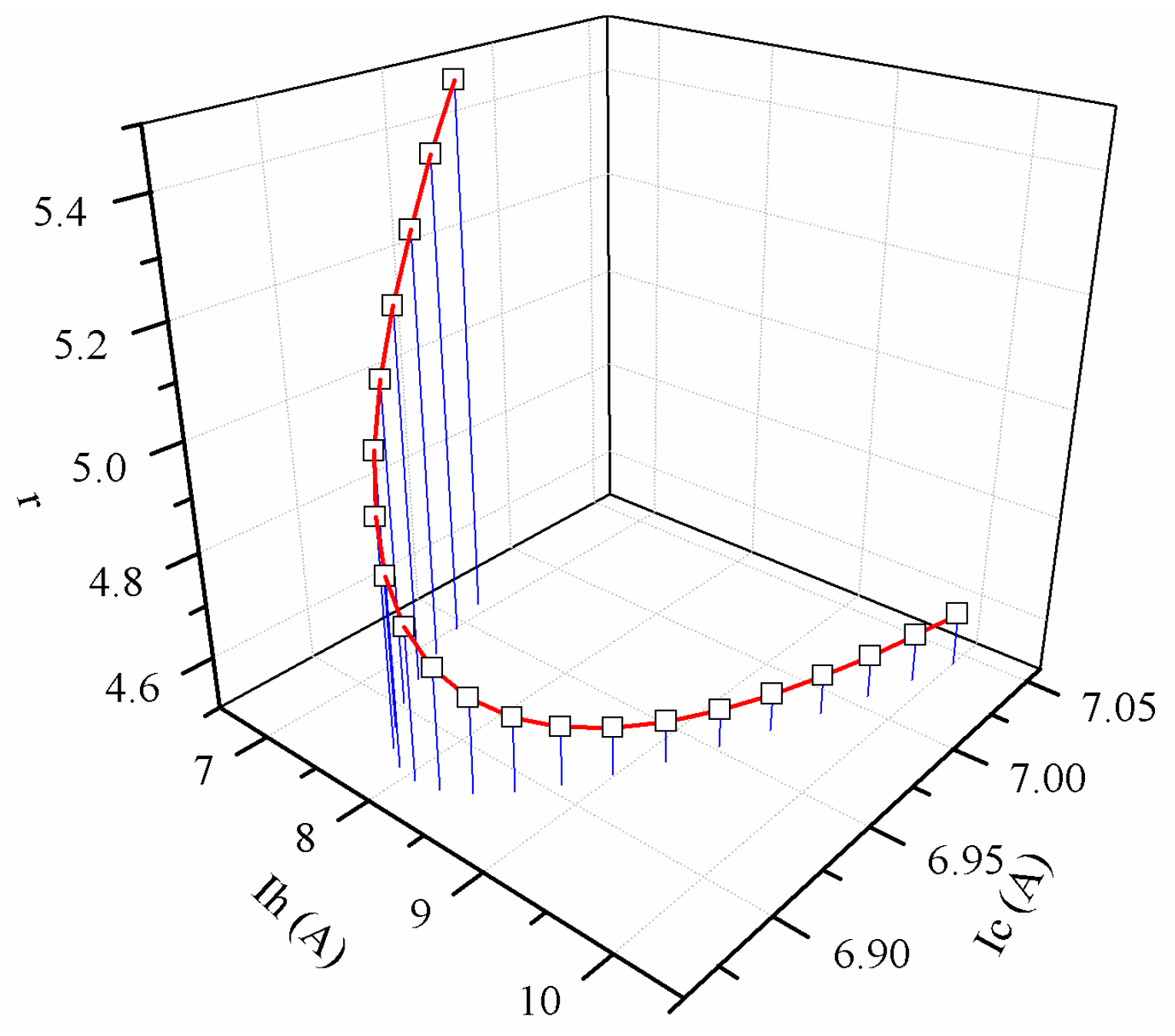
A satellite orbit constellation design usually relies on the Walker approach or streets of coverage method.
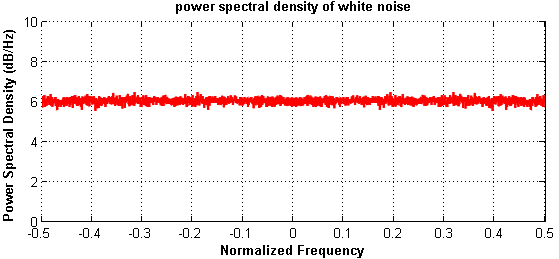
A constellation can offer unique capabilities that are often difficult to achieve through different means, for instance, enhanced temporal coverage.
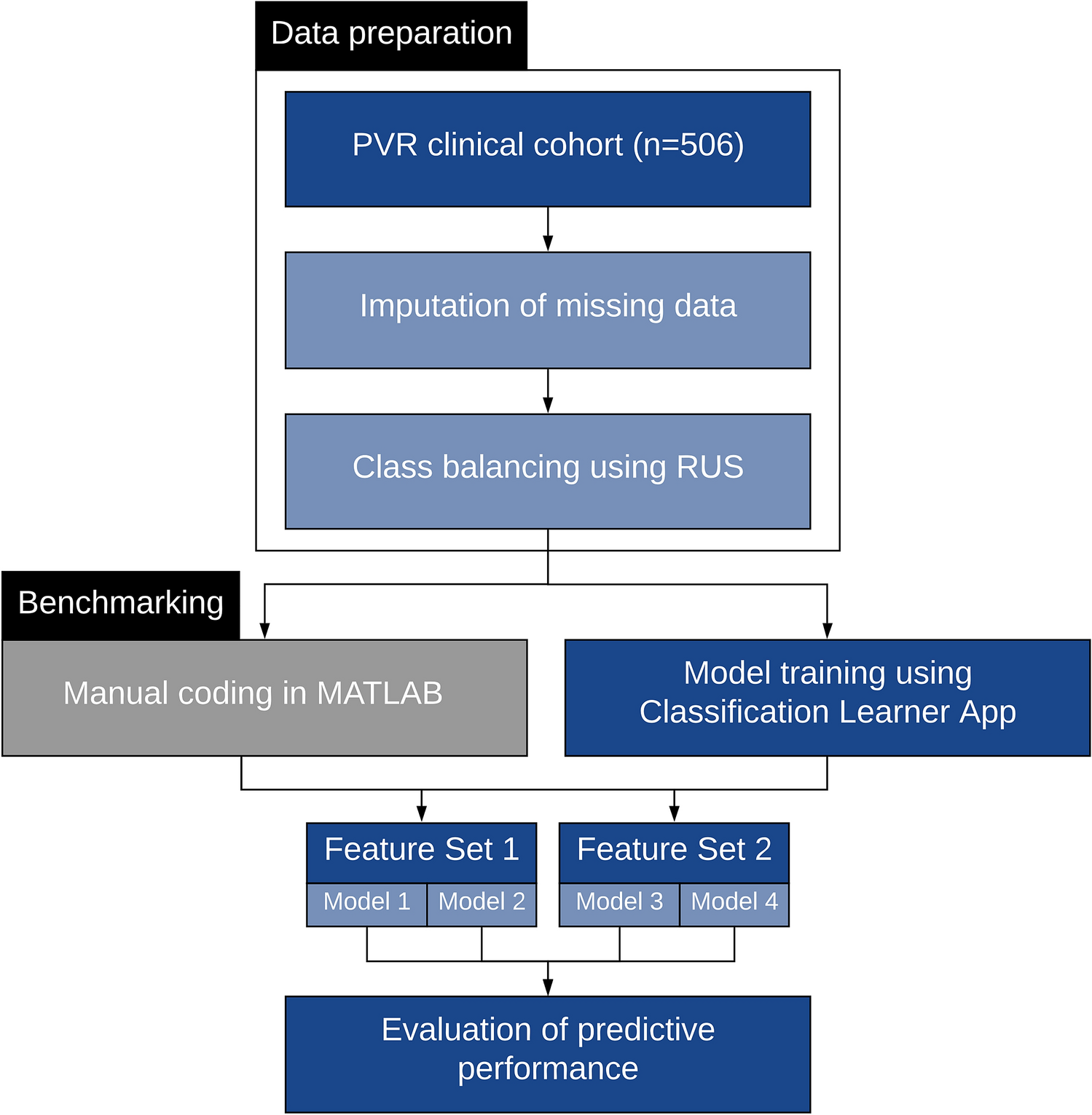
IntroductionĬompared to a single satellite, a constellation may provide better coverage and higher reliability under failure of some satellites, ensuring a higher rate of survival and mission success. To determine the best parametric operator combinations, the fitness value and the computational time from each study cases are compared. A semianalytical approach is implemented to speed up the guessing of initial orbital elements. Some efforts are made to improve the objective function and to minimize the computational load. A multiobjective optimization study is conducted in this study with percent coverage and revisit time as the two main parameters to analyze the performance of the constellation. From six orbital elements, five elements (semimajor axis, inclination, argument of perigee, longitude of ascending node, and mean anomaly) are varied as optimization design variables. A global optimization method, Genetic Algorithm (GA), is chosen due to its ability to locate a global optimum solution for nonlinear multiobjective problems. The constellation consists of nonsymmetric and circular Low Earth Orbit (LEO) satellites. A target area represented by a polygon defined by grid points is chosen instead of using a target point only. This paper focuses on maximizing the percent coverage and minimizing the revisit time for a small satellite constellation with limited coverage.
